Learning on Graphs: From Representation to Minimally Supervised
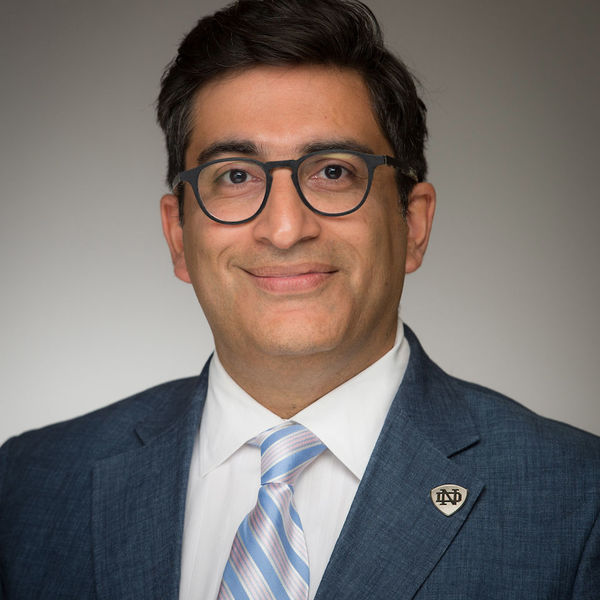
Nitesh Chawla
Nitesh Chawla, Ph.D. is the Frank M. Freimann Professor of Computer Science and Engineering at the University of Notre Dame and the Founding Director of the Lucy Family Institute for Data and Society. He is a Fellow of IEEE and Fellow of the Asia Pacific Association for Artificial Intelligence (AIAA). He is an expert in artificial intelligence, data science, and network science, and is motivated by the question of how technology can advance the common good through interdisciplinary research. He is the recipient of multiple awards for research and teaching innovation including outstanding teacher awards (2007 and 2010), a National Academy of Engineers New Faculty Fellowship, and a number of best paper awards and nominations. He also is the recipient of the 2015 IEEE CIS Outstanding Early Career Award; the IBM Watson Faculty Award; the IBM Big Data and Analytics Faculty Award; the National Academy of Engineering New Faculty Fellowship; and the 1st Source Bank Technology Commercialization Award. He is also a Fellow at the Kellogg Institute for International Studies; the Kroc Institute for International Peace Studies; the Liu Institute for Asia and Asian Studies; the Pulte Institute for Global Development; and the Reilly Center for Science, Technology, and Values. He is the co-founder of Aunalytics, a data science software and cloud computing company.
Graphs are ubiquitous due to their applicability of capturing the data attributes of complex systems, whether in social and information systems, chemical reactions, or healthcare applications. These graphs are themselves complex and heterogeneous with multi-modal, dynamic, and in some cases “small” data. In this talk, I will present our research on representation learning methods for heterogeneous data (going from shallow to deep embedding), graph learning algorithms for temporal and dynamic data, and few shot learning to enable minimally supervised learning in the presence of limited annotated data. I’ll share use cases and applications stemming from social systems, healthcare, and chemical synthesis.