Comparing Probability Distributions with Conditional Transport
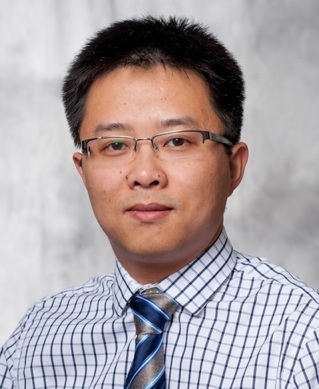
Mingyuan Zhou
Mingyuan Zhou is an Associate Professor of Statistics in the McCombs School of Business at the University of Texas at Austin. He received his Ph.D. in Electrical and Computer Engineering from Duke University in 2013. His research lies at the intersection of machine learning and Bayesian statistics, covering a diverse set of research topics in statistical theory and methods, probabilistic modeling, statistical inference for big data, and deep learning. He is currently focused on advancing both statistical inference with deep learning and deep learning with probabilistic methods. He has served as the 2018-2019 treasurer of the Bayesian Nonparametrics Section of the International Society for Bayesian Analysis, and as (senior) area chairs for leading machine learning conferences, including NeurIPS, ICML, ICLR, and AAAI.
To measure the difference between two probability distributions, we propose conditional transport (CT) as a new divergence and further introduce amortized CT (ACT) to make it amenable to implicit distributions and stochastic gradient descent based optimization. Equipped with two navigators that amortize the computation of conditional transport plans, ACT comes with unbiased sample gradients that are straightforward to compute. When applied to train a generative model, ACT is shown to strike a good balance between mode covering and seeking behaviors and strongly resist mode collapse. On a wide variety of benchmark datasets for generative modeling, substituting the default statistical distance of an existing generative adversarial network with the transport cost under ACT is shown to consistently improve the performance.