Towards Robustness Against Natural Language Adversarial Attacks
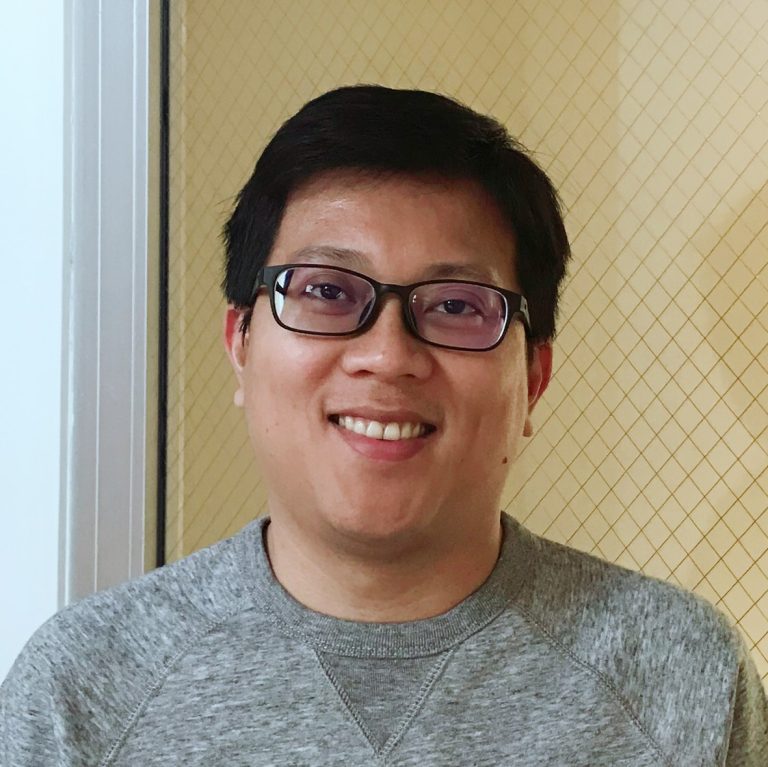
Anh Tuan Luu
Luu Anh Tuan is currently a postdoctoral fellow at the Computer Science and AI Laboratory, MIT and also a NLP research scientist at VinAI since May 2020. He will join School of Computing, NUS as an Assistant Professor next Spring. Tuan received his Ph.D. degree in computer science from NTU in 2016. His research interests lie in the intersection of Artificial Intelligence and NLP. He has published over 40 papers on top-tier conferences and journals including NeurIPS, ACL, EMNLP, KDD, WWW, TACL, AAAI, etc. Tuan also served as the Senior Area Chair of EMNLP 2020, Senior Program Committee of IJCAI 2020, and Program Committee member of NeuIPS, ICLR, ACL, AAAI, etc.
Recent extensive studies have shown that deep neural networks (DNNs) are vulnerable to adversarial attacks, e.g., minor phrase modification can easily deceive Google’s toxic comment detection systems. This raises grand security challenges to advanced NLP systems such as malware detection and spam filtering, where DNNs have been broadly deployed. As a result, the research on defending natural language adversarial attacks has attracted increasing attention. In this talk, we will first start with an introduction with different types of natural language attacks. We then discuss recent studies on natural language defense and their shortcomings. At the end of the talk, we introduce a novel Adversarial Sparse Convex Combination (ASCC) method that models the attack space as a convex hull and leverages a regularization term to enforce the perturbation towards an actual attacks, thus aligning our modeling better with the discrete textual space. Based on the ASCC method, we further propose ASCC-defense, which leverages ASCC to generate worst-case perturbations and incorporate adversarial training towards robustness. Ultimately, we envision a new class of defense towards robustness in NLP, where the obtained robustly trained word vectors can be plugged into a model and enforce its robustness without applying any other defense techniques.