An Integrated Framework for Controllable Text Generation
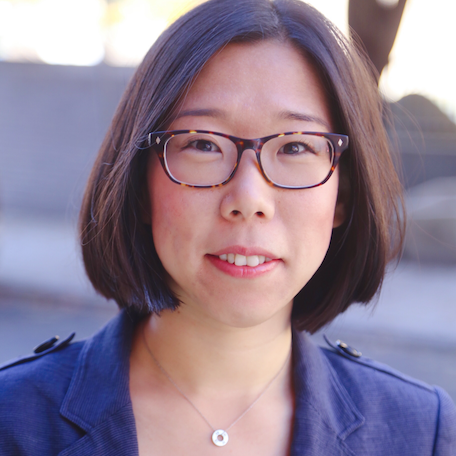
Wei Xu
Wei Xu is an assistant professor in the School of Interactive Computing at the Georgia Institute of Technology, where she is also affiliated with the new NSF AI CARING Institute and Machine Learning Center. She received her Ph.D. in Computer Science from New York University and her B.S. and M.S. from Tsinghua University. Xu’s research interests are in natural language processing, machine learning, and social media, with a focus on text generation, stylistics, robustness and controllability of machine learning models, and cross-lingual transfer learning. She is a recipient of the NSF CAREER Award, CrowdFlower AI for Everyone Award, Criteo Faculty Research Award, and Best Paper Award at COLING’18. She has also received funds from DARPA and IARPA. She is an elected member of the NAACL executive board and regularly serves as a senior area chair for AI/NLP conferences.
Natural language generation is an increasingly popular area for the application of deep learning techniques. In this talk, I will be discussing how we have tackled some of the longstanding challenges associated with this field, including the lack of interpretability and controllability in neural generation models, and the lack of task-specific training data and reliable evaluation methods. To achieve these goals, we have developed a new framework that consists of four major components: (1) high-quality data construction for real-world applications, such as text simplification and scientific writing; (2) controllable neural generation models; (3) interactive annotation interfaces; and (4) a redesigned and more reliable evaluation methodology. In particular, I will highlight our recent work on LENS, a learnable evaluation metric capable of differentiating between GPT-3.5 types of models that are highly competitive and perform at levels close to humans. LENS correlates better with human judgments than existing metrics, such as SARI and BERTScore, for evaluating text generation outputs. Moreover, LENS can serve as an effective learning objective in Minimum Bayes Risk (MBR) Decoding and Minimal Risk Training (MRT), which allows for the creation of state-of-the-art neural generation models that outperform fine-tuned large language models.